Developing Methodologies to Model Large and Complex Biomedical Data to Improve Health Outcomes
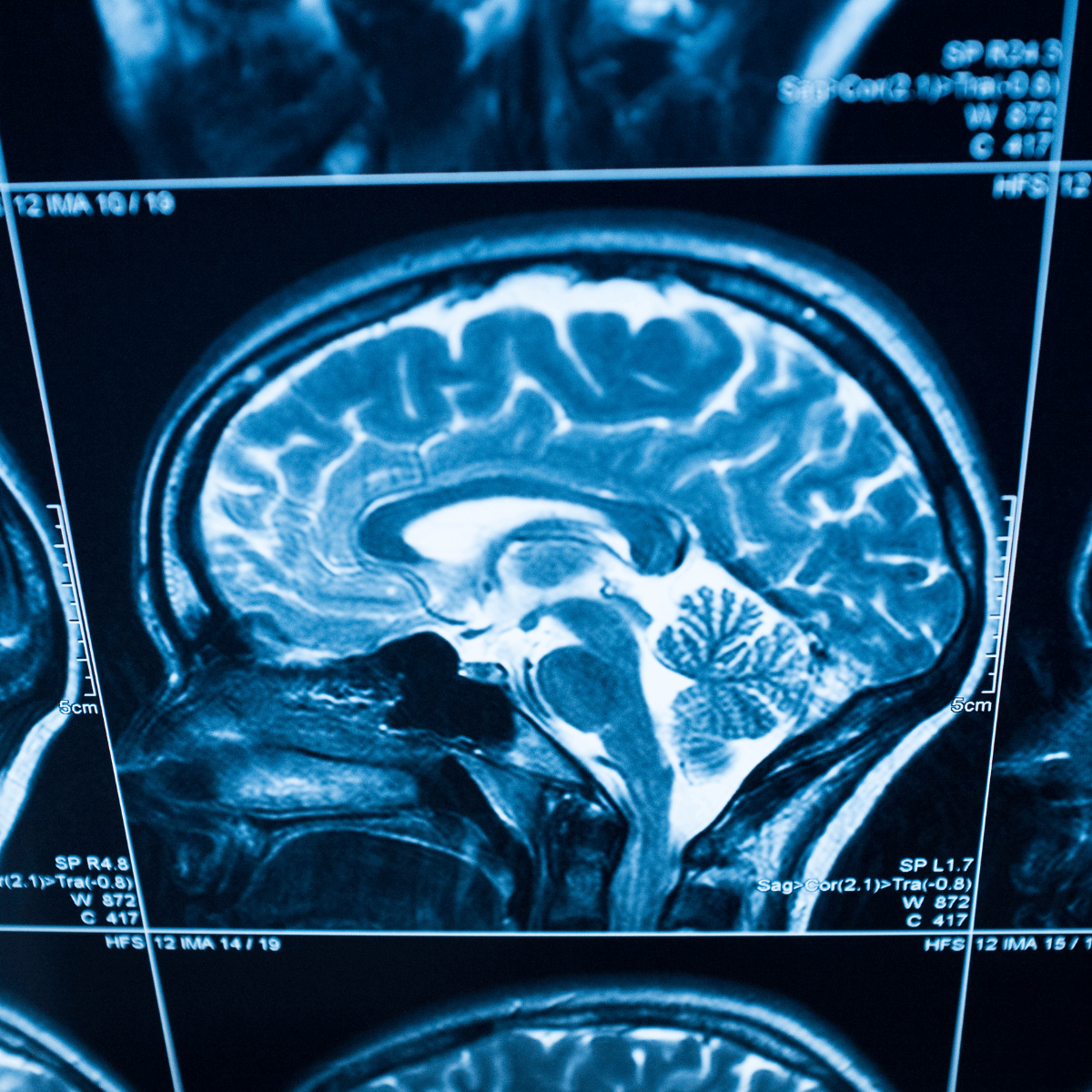
Our research group focuses on developing effective and impactful methodologies to
model large and complex biomedical data such as imaging and genomic data generated
by modern high-throughput technologies to have better insight into the disease
mechanism and improve the related health outcomes. We also closely collaborate with
basic science researchers, clinicians and epidemiologists to apply our method to solve
real-world biomedical problems.
Department: Epidemiology and Biostatistics
Room Number: SPH 2234M
Director: Tianzhou "Charles" Ma (UMD), Shuo Chen (UMB)
Office Phone Number: (301) 405-6421
Email: umdbright@gmail.com
We are a group of biostatisticians and bioinformaticians from the University of Maryland. Our research has focused on modeling large and complex biomedical data such as imaging and genomic data generated by modern high-throughput technologies. We aim to develop effective and impactful methodologies to have a better insight into the disease mechanism and improve the related health outcomes. We are actively seeking collaboration with biologists, clinicians, epidemiologists and researchers in all relevant fields.
For more details, please visit our website at www.umdbright.com.
Shuo Chen
Tianzhou (Charles) Ma
Qiong Wu
Yifan Yang
Yunjiang Ge
Tong Lu
Zhiwei Zhao
Eshetu Tefera
Song Liu
Hongjie Ke
Chen Mo
Jingtao Wang
Zhenyao Ye
Boao Zhao
Travis Canida
Hwiyoung Lee
NEUROIMAGING ANALYSIS:
Developing statistical models to handle the high-dimensional imaging variables with a complex covariance structure that is related to the spatiotemporal information for the group-level association analysis and inference. Developing machine learning models for individual-level diagnosis and prognosis.
GENETICS AND GENOMICS:
Developing rigorous and impactful statistical and bioinformatics methods tailored to the analysis of high-throughput genetic and genomic data in biomedical, epidemiological and clinical studies. for biomarker discovery, prognosis research and network analysis, etc.
INTEGRATIVE ANALYSIS:
Developing novel meta-analysis and integrative analytic methods to jointly analyze multimodal omics and imaging data to unravel the disease mechanism and ultimately inspire new approaches for the prevention and treatment of disease. Specific fields of interest include imaging genetics research for brain-related diseases, multi-level omics data integration in cancer application.
^: co-first author; *: corresponding author; students or trainees underlined
NEUROIMAGING ANALYSIS:
Wu Q, Huang, X., Culbreth, A., Waltz, J., Hong, L. E. and Chen, S* (2021). Extracting Brain Disease-Related Connectome Subgraphs by Adaptive Dense Subgraph Discovery. Biometrics, in press.
Ge, Y., Hare, S., Chen, G., Waltz, J. A., Kochunov, P., Elliot Hong, L. and Chen, S*. (2021). Bayes estimate of primary threshold in clusterwise functional magnetic resonance imaging inferences. Statistics in Medicine, in press.
Wu Q, Ma T, Liu Q, Milton D, Zhang Y and Chen, S*. (2021). ICN: extracting interconnected communities in gene co-expression networks. Bioinformatics, in press.
Chen, S., Bowman, FD. and Xing, Y. (2020). Detecting and Testing Altered Brain Connectivity Networks with K-partite Network Topology. Computational Statistics and
Data Analysis, 141, 109-122.
Chen, S., Xing, Y., Kang, J., Kochunov P. and Hong, E.L. (2020). Bayesian Modeling of Dependence in Brain Connectivity Data. Biostatistics, 21 (2), 269-286.
Chen, S., Kang, J., Xing, Y., Zhao, Y. and Milton, D. (2018). Estimating Large Covariance Matrix with Network Topology for High-dimensional Biomedical Data. Computational Statistics and Data Analysis, 127, 82-95.
GENETICS AND GENOMICS:
Ye Z^, Ke H^, Chen S, Cruz-Cano R, He X, Zhang J, Dorgan J, Milton D and Ma T*. (2021). Biomarker categorization in transcriptomic meta-analysis by concordant patterns with application to Pan-cancer studies. Frontiers in Genetics, 12.
Saegusa T, Zhao Z , Ke H , Ye Z, Xu Z, Chen S and Ma T*. (2021). Detecting survival-associated biomarkers from heterogeneous populations. Scientific Reports, 11(1): 1-12.
Ma T, Ren Z and Tseng GC. (2020). Variable screening with multiple studies. Statistica Sinica, 30(2): 925–953.
Ma T^, Huo Z^, Kuo A^, Zhu L, ..., Song C and Tseng GC. (2019). MetaOmics - Comprehensive Analysis Pipeline and Web-based Software Suite for Transcriptomic Meta-Analysis. Bioinformatics, 35 (9), 1597-1599.
Ma, T., Liang, F. and Tseng, GC. (2017). Biomarker detection and categorization in ribonucleic acid sequencing meta-analysis using Bayesian hierarchical model. Journal of the Royal Statistical Society: Series C. 66(4): 847-867.
Ma, T., Liang, F., Oesterreich, S. and Tseng, GC. (2017). A joint Bayesian modeling for integrating microarray and RNA-seq transcriptomic data. Journal of Computational Biology. 24(7): 647-662.
INTEGRATIVE ANALYSIS:
Ye Z^ , Mo C^, Liu S^, Hatch K, Gao S, Hong E, ..., Kochunov P*, Chen S* and Ma T*. (2021). White matter integrity and nicotine dependence: evaluating vertical and horizontal pleiotropy. Frontiers in Neuroscience, 15.
Mo C^, Ye Z^ , Ke H^ , Lu T, Canida T, ..., Hong E, Kochunov P, Ma T* and Chen S*. (2021). A new Mendelian Randomization method to estimate causal effects of multivariate brain imaging exposures. Pacific Symposium on Biocomputing (PSB) 2022,
Accepted.
Wang J, Kochunov P, Sampath H, Hatch KS, Ryan MC, Xue F, Neda J, Paul T, Hahn B, Gold J, Waltz J, Hong LE, Chen, S*. (2021). White matter brain aging in relationship to schizophrenia and its cognitive deficit. Schizophr Res, 230:9-16.
For a complete list, please visit our group’s website at www.umdbright.com or the Google Scholar pages of the co-directors:
Visit the Google Scholar Page for Dr. Shuo Chen.
Visit the Google Scholar Page for Dr. Tianzhou (Charles) Ma.